Team
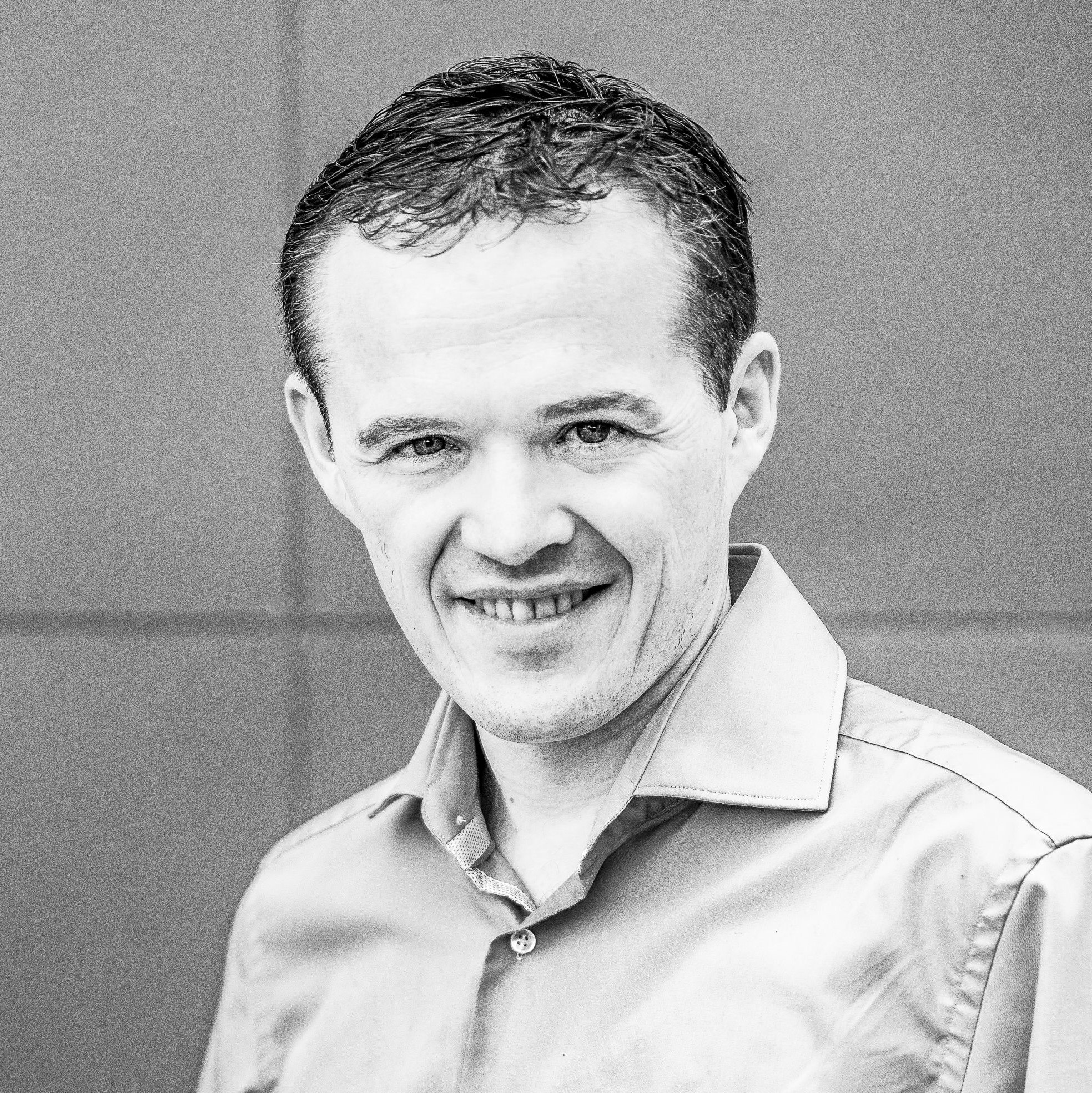
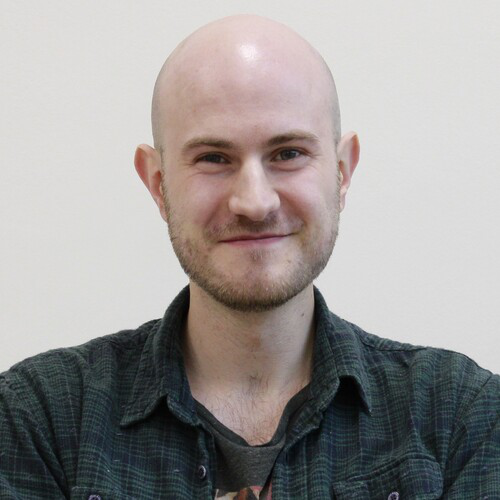
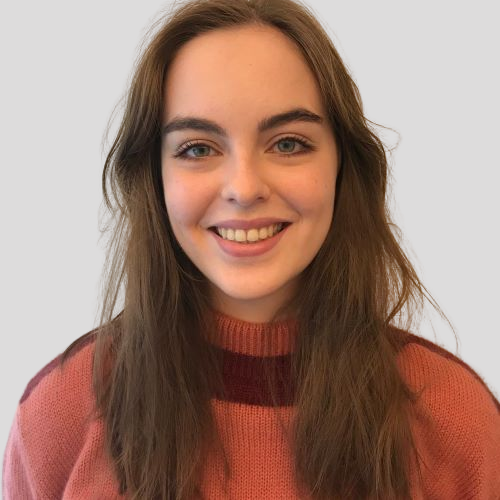
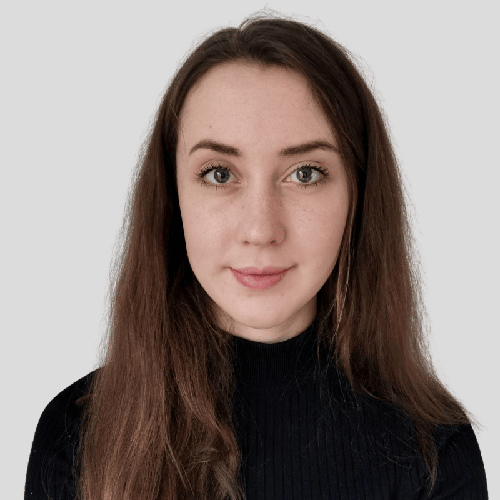
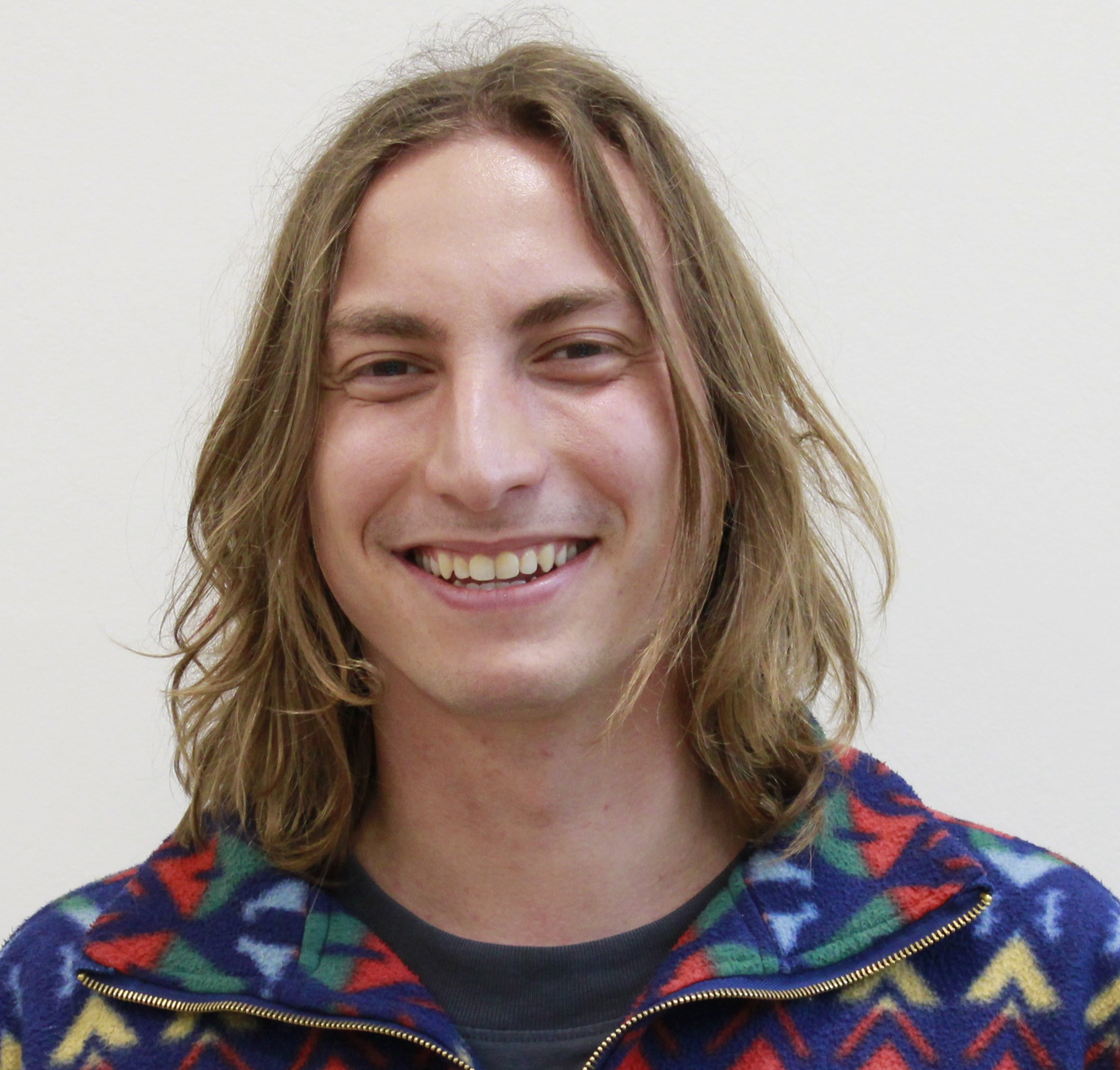
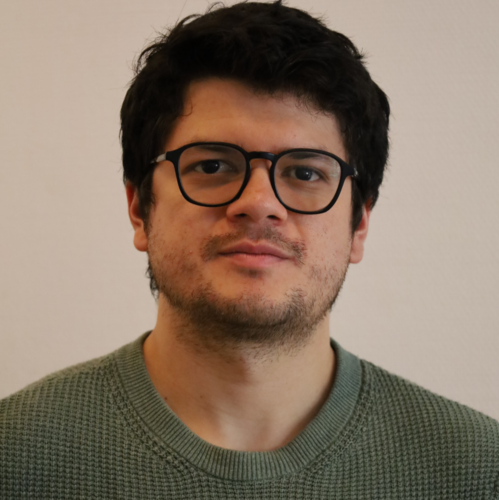
Our Former Members
Christina Papagiannopoulou Dimitris Iliadis Friederike Mey Jim Clauwaert Laure Van Den Bulcke Michiel Stock Nicolas Dewolf Peter Rubbens Thomas MortierJoin Us
If you are looking for a PhD or Postdoc position,
please contact Willem Waegeman.
Funding
Our work is made possible by funding from several organizations.
Thesis Students and Their Topics
Our group has had the privilege of guiding numerous thesis students through their research projects. Below is a list of these students, their thesis topics, and the year of their thesis:
(2024 – 2025)
- R. Claeys - Variational inference for DTI
- W. Welvaert - End-to-end de novo metabolomics
(2023 – 2024)
- S. Jimenez - Disentangling uncertainties in Machine Learning: A comparative approach.
- J. Cueva Villavicencio - Novel neural networks for bacterial species prediction from MALDI-TOF data
(2022 – 2023)
- J.-H. Nowé - Countering illegal timbering by using multimodal machine learning
- N. Tourne - Two-branch neural networks for predicting protein-DNA interaction
(2021-2022)
- Y. Van Laere - Detection of 5mC modification in Nanopore sequencing data using deep learning
- V. Moortgat - On Calibration of Probabilistic Multi-Class Classifiers with Hierarchical Factorization
(2020 – 2021)
- E. Lorrez - Quantifying the environmental controls on African tropical forest dynamics through using a Granger causality framework
- L. Theunissen - A comparison of flat and hierarchical classification for automatic annotation of single-cell transcriptomics data
- T. Willaert - Improving classification by rejection of high epistemic uncertainty points
- K. Zhang - Drug-target interaction prediction using multi-target prediction methods
(2019 – 2020)
- L. Davey - Artificial neural networks to uncover features in promoter sequences responsible for nonorthogonality in E. Coli
- B. De Saedeleer - Combatting illegal timber trade using chemical fingerprints: the power of mathematics and mass spectrometry
- L. Pollaris - Protein secondary structure prediction using transformer networks
- G. Tjon - Automative drinking water monitoring using flow cytometry data
- B. Verfaillie - Pattern recognition in raman spectroscopy data for a faster labelling of subjects in multiple domains
(2018 – 2019)
- K. D'haeyer - Towards a data-driven identification of the microbial "Rammanome"
- B. De Clercq - Forecasting tidal surge in the Lower Sea Scheldt using machine learning techniques
- G. De Clercq - Deep learning for classification of DNA functional sequences
- N. Goeders - Fight the illegal wood trade through chemical fingerprints: The power of mathematics and mass spectrometry
- S. Top - Vertical farming of lettuce: the influence of rhizosphere bacteria and substrates
- M. V. Haeverbeke - Detection of m6a modifications in native RNA using Oxford Nanopore Technology
(2017 – 2018)
- A. De Graeve - Detecting climate drivers for vegetation extremes
- R. Ingels - Understanding vegetation anomalies with machine learning methods
- M. Misonne - Prediction of RNA polymerase-DNA interactions in Escherichia Coli
- T. Vanlerberghe - Hierarchical multi-label classification of food products
(2016 – 2017)
- L. Bodyn - Exploration of deep autoencoders for collaborative filtering on cooking recipes
- S. Decubber - Spatiotemporal optimization of Granger causality methods for climate change attribution
- J. Heyse - Development and application of single-cell analysis tools for the study of sympatric bacterial populations
- T. Mortier - Modeling of climate-vegetation dynamics using machine learning techniques in a non-linear Granger causality framework
- D. Schaumont - Een integratieve benadering gebaseerd op random forest voor de verbeterde predictie van exon-intron juncties
- X. Yin - Discovering relationships in climate-vegetation dynamics using dynamic feature selection techniques
- C. Zhang - Visualization and unsupervised learning of flow cytometry data for bacterial identification
(2009 – 2016)
- F. Ramon - A data-driven analysis of ingredients in cooking recipes
- L. Tilleman - In silico engineering van cytochroom P450 via machine learning technieken
- J. De Reu - Analyse van voorspellingsmodellen voor aardappelziekten en hun toepasbaarheid in Vlaanderen
- W.K. Tsang - Assessing pathogen invasion based on community evenness and metabolic similarity
- M. De Clercq - Prediction of ingredient combinations using machine learning techniques
- A. De Paepe - The prediction of interaction between mRNA and miRNA using machine learning techniques
- M. Stock - Learning pairwise relations in bioinformatics: three case studies
- J. Vandepitte - Voorspellen van Fusarium spp. aanwezigheid en DON concentraties in wintertarwe met machine learning technieken